NOTE: I HAVE ATTACHED THE PAPER OUTLINE TO THIS POST. PLEASE USE THE UPLOADED FILE TO COMPLETE THE WORK.
- 8-10 pages (double-spaced) Times New Roman 12 pt font.
- Must have Abstract, Table of Contents, Introduction, Conclusion and section headings
- Use at least five references outside of your textbook (you may use your textbook too, but are not required to).
- In addition to the required number of pages for the assignment, you must also Include a reference page (bibliography), written in APA style and a title page. Be sure to give all of your papers a descriptive title!
- You must submit a rough draft at the end of Week 5. This is to be a complete paper, meeting the page requirements – not a partially completed paper. Points will be deducted for short or incomplete papers. Your rough draft will not be graded by the rubric, but helpful feedback will be provided to indicate where you are falling short.
1
2
Enhancing Database Security through Machine Learning: Anomaly Detection and Response
Prince Boateng
Instructor:
American Military University
Class:ISSC290
6/16/2024
More sophisticated security measures must be in place as the nature of cyber threats continues to change in favor of complexity, particularly for highly valuable database systems. Efforts to avert new or even complex hazards are hardly supported by basic precautionary measures. Given these conditions, this research article suggests utilizing machine learning (ML) to enhance security by means of anomaly identification and automated reactions to such anomalies within a database system. To detect patterns and indicators of a security breach, large amounts of data can be analyzed using models that incorporate machine learning. The discussion that follows offers many MLALGs in this regard. The benefits of real-time anomaly detection are accessed in order to evaluate real-time threat handling with minimal impact on the database.
In 2020, Gupta and colleagues introduced taxonomy of machine learning models utilized in safe data analytics, highlighting their suitability and constraints for threat discovery and mitigation. The authors highlight the necessity of creating a suitable threat model that will show how cyber threats are always changing and taking on new forms, which is why they advocate for ML-based security solutions that are intelligent and adaptive. Similarly, when employing machine learning models, Xue et al. (2020) talked about security issues and potential solutions. The authors emphasize that evaluating the security of ML-models and countermeasures against hostile attacks is a crucial area of focus for their research. Every one of these examples highlights the potential and needs for machine learning (ML)-based techniques in database security that aim to increase efficiency and capability.
The efficiency of ML-based IDS on imbalanced datasets is the subject of an article by Karatas et al. (2020), which is followed by this discussion. According to this paper, optimized machine learning models will aid in danger identification, lower false alarms, and preserve accessibility and dependability in database systems.
High-level Outline
i.d
Issues in implementing security some databases
Challenges associated with conventional approaches to security.
Essential Machine Learning Concepts and Choices for Anomaly Detection
Objectives and Significance of the Study
ii. Literature Review
A Brief Insight into Machine Learning in Cyber Security,Gupta et al. (2020)
Security Threats and Risk Mitigation Algorithms in Machine Learning (Xue et al., 2020)
The Effectiveness of IDS ML in the Creation and Training of Data Sets,Karatas et al., (2020)
iii. Methodology
Capture and pre-process end-user data
Artificial and live database transaction data.
Machine learning's farthest limit: coming out ahead
Supervised, unsupervised, and semi-sup
Training and Assessment Measures
Accuracy, detection speed, false positive rate
iv. Automated Response Strategies
Design of Automated Response Mechanism
access control, intrusion detection and alarm/notification
Integration with anomaly detection models
Evaluation of response effectiveness
v. Performance Impact Analysis
Securing database performance assessment by machine-learning-based security approaches
Trade-offs between security and performance
Examples or case studies of lived experiences
vi. Discussion
Implications of the Findings
Compare to a regular protocol for security
Challenges and limitations
vi. Conclusion
Summary of key achievements
Future research avidness
Concluding remarks
References
Gupta, R., Tanwar, S., Tyagi, S., & Kumar, N. (2020). Machine learning models for secure data analytics: A taxonomy and threat model. Computer Communications, 153, 406-440. https://doi.org/10.1016/j.comcom.2020.02.008
Karatas, G., Demir, O., &Sahingoz, O. K. (2020). Increasing the performance of machine learning-based IDSs on an imbalanced and up-to-date dataset. IEEE access, 8, 32150-32162. https://doi.org/10.1109/ACCESS.2020.2973219
Xue, M., Yuan, C., Wu, H., Zhang, Y., & Liu, W. (2020). Machine learning security: Threats, countermeasures, and evaluations. IEEE Access, 8, 74720-74742. https://doi.org/10.1109/ACCESS.2020.2987435
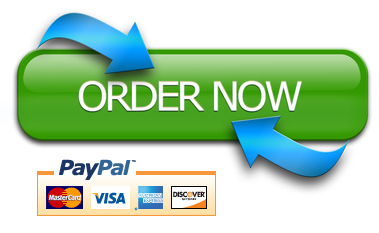